December 12, 2023 – Online
The international working group, formed in the frame of the CHAD project, had its eighth meeting on 12 December 2023. The topic was the connection between hate speech and artificial intelligence (AI) in the online sphere. The meeting focused on how different methods, be that activism, regulations, or specific detector programs, can prevent, protect from, and mitigate the harms of online hate speech.
Four presentations took place at the meeting. The first was by Frederike Kaltheuner, a tech policy expert, who detailed the current challenges of regulating and understanding the nature of online discrimination and its relationship to AI. Danguolė Kalinauskaitė, a lecturer and Justina Mandravickaité, a researcher from Vytautas Magnus University, Lithuania, gave the second presentation showcasing their #BeHate-Free project (also co-author Prof. Dr. Tomas Krilavičius, Dean of the Faculty of Informatics, Vytautas Magnus University, Lithuania), which saw them developing particular algorithms to detect and identify hate speech on the internet. The third presenter, Xavier Brandao, director of #jesuislà, a small French NGO advocating for stricter Big Tech regulations, gave an overview of the organisation’s history, projects, achievements, and prospects. The last presenter, Emillie V. de Keulenaar, a PhD researcher at the University of Groningen and consultant for the UN DPPA Innovation Cell, offered a brief look into how Large Language Models (LLMs) can reproduce and maintain historical conflicts and intolerance.
Summary of the Presentations
Hate Speech and AI – Bias Amplification
Frederike Kaltheuner began her presentation by stressing that this is a crucial moment regarding AI regulation and hate speech awareness online. She laid down three main issues that will likely determine how AI can influence online hate speech.
The first is the changing nature of discrimination and exclusion as a result of hate speech entering the digital arena and obsolete thinking that freedom of expression means the right to speak. In turn, the moment someone engages in hate speech it restricts the victims’ ability to effectively use their right to freedom of expression – this has to be in the law. The real danger is the pace and sophistication of how discrimination and excursion are being “baked into the technical infrastructure”. This phenomenon entails that as platforms and their infrastructure grow, so does the scale of discrimination, exclusion, and bias, making it much harder to tackle them on time. Lastly, she shed light on how even with good intentions, systems can discriminate, and due to such a massive amount of data and scale of growth, it takes months and sometimes years to detect such cases, also in part because it is immensely challenging to prove what counts as hate speech – this makes applying already existing legal frameworks even more difficult in the online space.
The second point is the relationship between AI, platforms, and discrimination and exclusion. All platforms are different in structure, infrastructure, content, etc. This inherently makes regulating them increasingly troublesome. Kaltheuner introduced the two sides of platforms, the front and back end. The back end is the technical infrastructure: what can or cannot be shown, what does or does not get amplified, for instance, banner ads, which can be excluded; it also includes recommending, which by default is not possible to be neutral. The front end is the content itself, of users and ads moderated by the platform. While hate speech most often occurs in the form of content, it is the back end that should be adequately regulated to stop such content from arising.
Lastly, the challenges of platform accountability and regulation were presented. The case of platform accountability is portrayed as a double-edged sword: on the one hand, under-enforcement, while on the other, over-blocking. This conundrum is exacerbated by the visible unreadiness of AIs to make nuanced distinctions, for example, between an ironic and harmful comment. Nonetheless, the conditions in which human moderators have to work are appalling. Platform accountability’s double-edged sword is multifaceted, as next to the aforementioned aspect, moderation is also a dilemma in this regard: not sufficient enough AIs or a nuanced human workforce in inhumane conditions.
The main argument for platform regulation is that platform self-regulation does not work – this is enforced by such debacles as the Twitter ownership change, which showed the fragility of a platform’s reliability. A recent highlight is the Digital Services Act (DSA) in the EU, and although it is not perfect, it is the first of its kind to force platforms to be more transparent and accountable. Yet, Kaltheuner warned that the DSA will only fulfil its purpose if it is enforced by member states. A crucial point of contention regarding the DSA is that it is focused too much on hate speech and misinformation while giving significant tools in the hands of states to act against such problems, missing the fact that governments can also be the perpetrators of these acts.
Hate speech and AI – AI literacy, the #BeHate-Free project
The #BeHate-Free project sprung out of a collaboration between Vytautas Magnus University, the Lithuanian Human Rights Center, the European Foundation of Human Rights, and the Department of National Minorities in the Lithuanian Government. Launched in 2021 and concluded in early 2023, the project was to address the challenges posed by hate speech by developing a prototype algorithm for hate speech detection. The initiative was made to educate and raise awareness amongst vastly diverse groups, including decision-makers, the criminal justice system, youth and youth workers, affected communities, and the general public.
Slowed down by the vagueness of hate speech and the lack of any general definition, the project’s first objective was to explore and identify linguistic forms of hate speech while working on options for automated detection. Several varying forms of hate speech were examined throughout different channels of social media, such as comments and posts. After establishing a specific framework of hate speech-allusive linguistic forms. The study began its second phase of exploring automated approaches, utilising deep learning and machine learning methods.
The project consisted of numerous different pathways, including the translation of several movies distributed to students and professionals to promote discussions on how to communicate with young people about hate speech. Special training programs were introduced for youth workers, workshops were developed for teachers and youth workers to be aware of topics such as online hate speech and safety, thus enabling them to identify and report hate speech.
One of the main challenges of the project was to tackle the vagueness of hate speech, making it more tangible and, therefore, more recognisable. For this goal, the project came up with criteria: the presence of a target, promotion of violence or hatred towards a specific group or individual, attacking or demeaning of groups or individuals, and it often has some form of humour included, for instance, sarcasm, further complicating identification.
To train algorithms to detect and identify online hate speech, the project needed a large and relatively comprehensive dataset. The creation of such a sample was a threefold process through data mining, annotation, and pre-processing. Data mining meant the crucial selection of news portals’ and online platforms’ contents, while annotation involved categorising content into hate speech, non-hate speech, and offensive speech, pre-processing aimed at mitigating the challenges posed by language nuances, and intentional misspellings.
The initial dataset overview revealed non-hate content to be the largest proportion at 60.7%, offensive speech stood at 31%, and hate speech, although a seemingly small amount at 8.26%, is anything but negligible, as the presenters warn many times that the harm caused by such content is immense. They found hate speech to centre on four main topics, in descending order: foreigners, LGBTQ issues, gender-related discrimination, and antisemitic content.
As the final step, artificial intelligence methods were employed to develop three deep learning models capable of classifying and detecting Lithuanian online content into the three defined speech categories (non-hate, offensive, and hate speech). Additional testing of these models used the Telegram platform to create a data pool. Furthermore, all three models with little variations showed the same proportions of the three kinds of speech in the dataset.
The project raised questions about the effectiveness of using AI to tackle online hate speech, emphasising the need to consider the harm caused beyond the technical capabilities of the models. The presenters emphasised both the effectiveness and usefulness of such models while admitting to the limitations and risk of letting algorithms categorise hate speech to some extent. Reflections were made on the potential application of AI models to categorise misinformation in articles, with considerations for language-specific datasets and the extent of training required.
The #BeHate-Free project was valuable on many levels, including the education of students on the topic, the training of teachers and youth workers, the creation of online speech classes, and the development of a dataset and three AI models to detect and identify such speeches. The project also holds potential future utilisation prospects of AI models to recognise misinformation in articles, but undoubtedly, more language-specific datasets are needed for such projects.
#jesuislà: Advocating for Big Tech regulation as a small NGO
Xavier Brandao, the director of #jesuislà, a small French collective of activists trying to counter hate and misinformation online, presented the group’s story and achievements, arguing that it is possible to attain objectives against Big Tech, even as a small NGO.
The association started as a Facebook group, where like-minded people were directly advocating with platforms through flagging, reporting, etc. It could be realised soon it yielded very little meaningful change and this realisation prompted the group to shift their focus to regulations instead of intra-platform advocacy. Their mission statement was to speak up against online hate while pushing for change in the Big Tech regulatory environment.
Initially, the group worked collaboratively with platforms, yet while they received grand promises, there were little palpable improvements from the companies’ sides. Therefore, the group joined a large NGO coalition, which gave them access to information, personnel, and expert help in the field. While some of the workings of the collective were coordinated to fit the grand agenda of defending people vs Big Tech. It also gave freedom to its member groups, who, in turn, could focus on their domestic issues and a myriad of other concerns.
Throughout the past years, #jesuislà has signed sixty open letters to various bodies, primarily supporting some form of regulatory action. Apart from constant online campaigns, the group developed a new directive: they would work as a bridge between European civil society, Brussels, and Paris to bring the knowledge and trends back to France.
The presentation stressed how the AI Act was created and what challenges EU lawmakers and NGOs faced. First, AI regulation is still in its baby shoes; there are many varieties, for example, low and high-risk AIs. It is an exceptionally complex topic, focusing on a different angle from the DSA, such as the usage of AI by law enforcement. The complexity of the issue only exacerbates the already hardly followable bureaucratic processes in Brussels, thus such groups like #jesuislà ought to simplify and educate the public on it. The nature of the AI Act and its surrounding drafting period meant that there was barely any buzz around it initially. Nonetheless, after early 2023 and the bombshell entry of public-use generative AIs, such as ChatGPT, turned more heads towards the topic and the dangers of such models. AI is not Skynet, but there are constant human rights violations committed by such algorithms on a technicality. The tremendous appeal of new openly accessible AIs implied that the AI Act would need to include and regulate generative and large AI models as well. The process seemed to be lengthy, stemming from the complexity of the case, yet on the 9th of November 2023, it was revealed that a few countries led by France wanted large foundation AI models (or general-purpose AIs) to avoid regulation, and instead, that platforms would ensure self-regulation for these models. This was a major shock to many experts and advocates of Big Tech regulation, who were trying to show how large models are even more dangerous due to more potential room for bias and the fact that many AI applications will be built on them. After a major pressure and media campaign by the NGO collective and other actors, notably in the culture sector, obligations for foundation models were kept in the AI Act, and France, who threatened to block the text, was isolated and finally voted in favour. The AI Act was adopted by the member states. The vote in the EU Parliament plenary will take place in April and the AI Act is expected to be definitely adopted then. The cooperation between all these diverging groups is essential to change. Xavier Brandao concluded by stating how 67% of French people are worried about AI; #jesuislà wishes to be a voice for these unheard people in Paris and Brussels.
How LLMs perpetuate historical intolerance: GPT, Google FLAN and the Nagorno-Karabakh war
In this presentation, instead of focusing on how to detect and identify, Emillie de Keulenaar presented hate speech as a product of histories of conflict – conflicts are training grounds for machine learning. Large Language Models (LLMs) are overwhelmingly trained on English language datasets, about 90% of all data – this means that the likes of ChatGPT and Google FLAN will have very particular knowledge, which is primarily based on English language literature. De Keulenaar introduced the following research question: how are historical conflicts reproduced by LLMs that speak minority languages? Focusing on autocompletion can give a clear idea of how the LLM has been trained.
The recent conflict in Nagorno Karabakh is functioning as the case study for the research – it works exceptionally well, as next to English, two local, smaller languages constitute the opposing sides of the clash. The brief overview of the results was that based on languages, the answer the LLM gives varies greatly in line with the general, often nationalistic sentiments ingrained in the nation and its language, thus reproducing conflicts. The question hence begs itself: why are LLMs’ answers in minority languages more discriminatory and politicised? The two possible explanations are: the first is Wikipedia, which is arguably the most easily accessible online knowledge site, however, it is crucial to note that it can be edited by almost anyone, especially in minority languages, where there is no abundance of sources for historical topics. Second is governmental sources, whose prominence in LLM datasets stems from the aforementioned lack of a wide array of perspectives in the language available to the model.
The presentation ended with three possible ways to remedy this problem: strengthening consensus viewpoints within LLM training datasets (such as Wikipedia), thereby producing “peacebuilding training datasets” with more balanced distribution of viewpoints, and designing alternative LLM prompt response formats for controversial questions, with for example responses that provide context and explanations to the many sides of a conflict.
This brief study showed that even now, in the very early stages of LLM management, there are already visible issues of discrimination and bias; this issue is even more pressing as ChatGPT and the like are becoming ever more popular and as they are being integrated into general internet usage, possibly at the expense of Wikipedia and other sites, making them highly likely to be the primary source of knowledge soon.
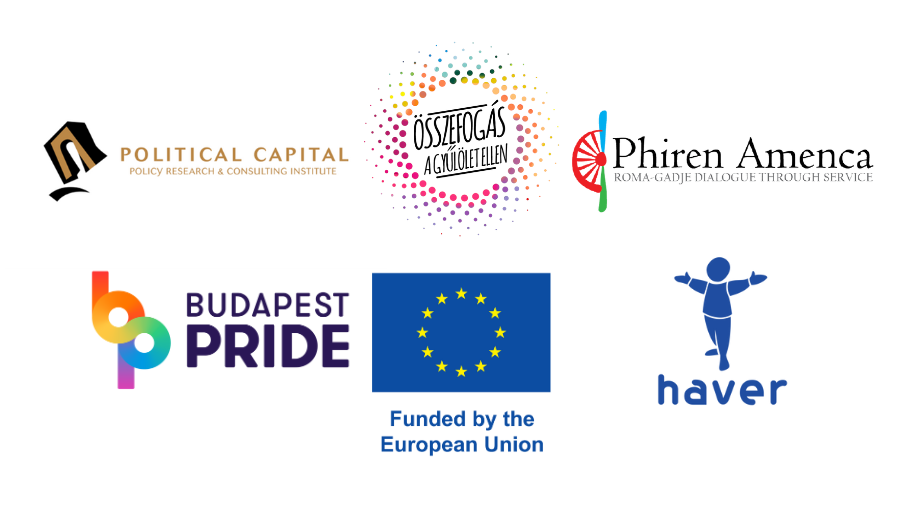
The „CHAD – Countering Hate Speech and Hurtful Speech against Diversity: Roma, LGBTIQ, Jewish and Migrant Communities” project (project nr. 101049309) is funded by the Citizens, Equality, Rights and Values Programme (CERV) of the DG Justice, European Commission and coordinated by RGDTS Nonprofit Llc. in partnership with Haver Informal Educational Foundation, Rainbow Mission Foundation and Political Capital. Views and opinions expressed are however those of the author(s) only and do not necessarily reflect those of the European Union or the European Commission. Neither the European Union nor the granting authority can be held responsible for them.